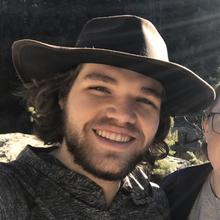
- Audiences
- Interests
- Keywords
- Types
2023 Prospective Student Visit Day and Graduate Research Symposium
Nov 3, 2023
01:30 PM - 05:00 PM
University Capitol Centre, 2520D
200 South Capitol Street, Iowa City, IA 52240
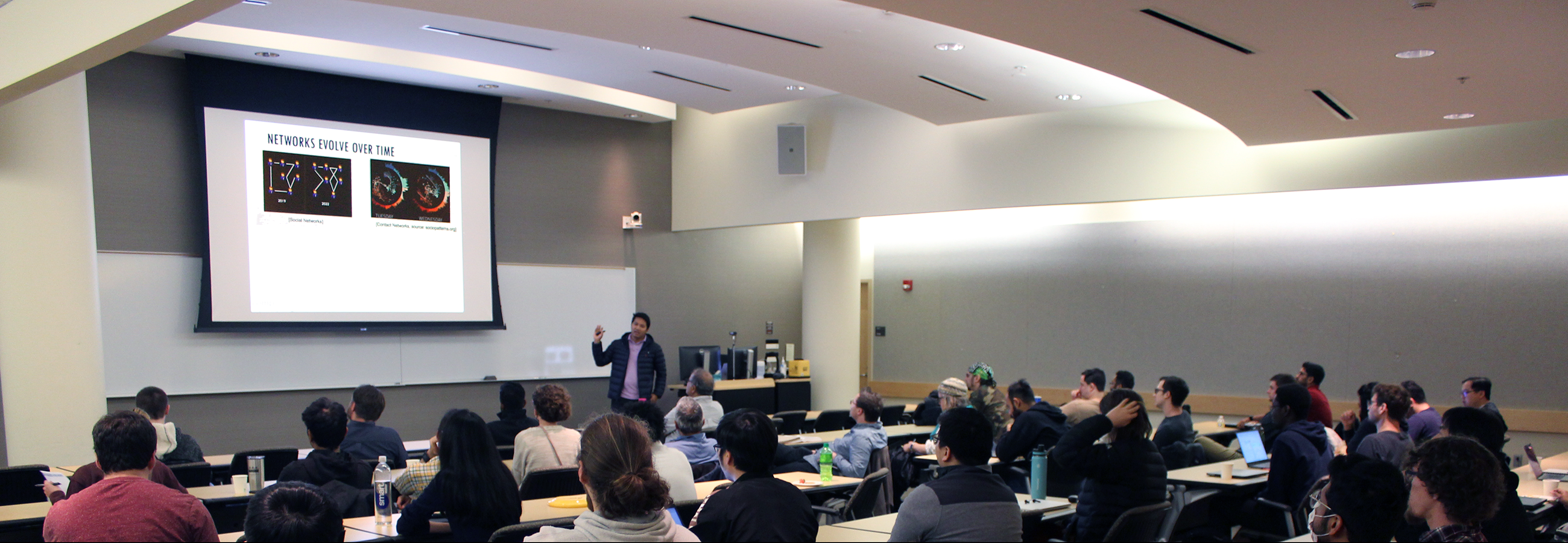
The Computer Science (CS) department welcomes prospective students interested in our graduate programs to our annual Prospective Student Visit Day on Friday, Nov. 3. We are looking for strong students with diverse backgrounds to join our MCS and PhD programs. A wide variety of research areas is represented by our world-class faculty including algorithms, computational epidemiology, distributed computing, human-computer interaction, machine learning, massive data algorithms and technology, mobile computing, networks, programming languages, text mining, security, and virtual reality. Write to our Graduate Program Administrator at cs-info@list.uiowa.edu if you'd like to visit.
An important part of the prospective Student Visit Day is the 9th Iowa Computer Science Graduate Research Symposium (2023), in which senior PhD students will present talks showcasing their current research. This will be followed by a keynote by a CS faculty member. Talks are intended for a wide audience with interest in CS, including CS juniors and seniors. The talks presented by current CS graduate students at the Symposium are excellent examples of the exciting CS research taking place here on the UI campus!
Schedule
All times CT [Conversion to your timezone possible here if needed]
Friday, Nov. 3, 2023 |
Morning Session (By invitation) |
|
Morning |
Overview of Graduate Programs by Professor and Director of Graduate Studies Steve GoddardShort Faculty Research Presentations by Professors Peng Jiang, Mehrdad Moharrami, Rishab Nithyanand, and Padmini Srinivasan |
|
Graduate Student Research Symposium Sessions |
||
1:30-3:25 p.m. |
Student Sessions |
|
1:30 p.m. |
Jeffrey Keithley, PhD Student Designing Near-Optimal Spatial Vaccine Allocation Strategies |
|
2 p.m. |
Yumna Anwar, PhD Student Audio-Based Cough Detection in Clinic Waiting Rooms |
|
3 p.m. |
Ingroj Shrestha, PhD Student Studying Bias in Large Language Models |
|
2:30 p.m. |
Maaz Bin Musa, PhD Student Unveiling the Digital Veil: Empowering Transparency and Accountability in Online Privacy |
|
3:25-4 p.m. |
Break |
|
4-5 p.m. |
Keynote |
|
Speaker: Professor Garrett Morris |
||
Speakers
Jeffrey Keithley
Title: Designing Near-Optimal Spatial Vaccine Allocation Strategies
Abstract:
As we have observed in the case of COVID-19 and the 2009 H1N1 pandemic, effective vaccines for an emerging pandemic tend to be in limited supply and must be allocated strategically. This vaccine allocation problem can be modeled as a discrete optimization problem and prior research has shown that this problem is computationally difficult (i.e., NP-hard) to solve even approximately. In our main theoretical result, we show that this hardness result can be circumvented, i.e., the problem becomes easier to approximately solve for diseases that have lower infectivity. We present our results in the context of a meta-population model, in which vaccines are allocated at a subpopulation level. In this setting, the vaccine allocation problem can be formulated as a problem of maximizing a function f defined over an integer lattice (i.e., integer vectors), subject to a budget constraint. We propose a simple, inherently parallelizable, greedy algorithm for this problem. We show the quality of approximation depends on a measure of how distant f is from being submodular (when the per-unit marginal gain decreases for each iteration of the algorithm), which we call the lattice submodularity ratio of f. We then experimentally demonstrate, for various natural formulations of f (e.g., total number of cases averted), that the lattice submodularity ratio of f depends on disease parameters of the underlying model. For less infectious diseases, the function f approaches submodularity, and the greedy algorithm provides a better approximation to the vaccine allocation problem. To experimentally demonstrate our methods, we build a state-level meta-population model (e.g., for Iowa) using human population and mobility datasets from FRED and SafeGraph. We construct a modular optimization code framework for meta-population models which supports the interchangeability of individual subpopulation disease models. Our experimental results confirm our theoretical results and show that the simple greedy algorithm is a scalable vaccine allocation algorithm that is near-optimal, especially for low-infectivity diseases.
3rd year PhD student | Advisors: Bijaya Adhikari; Sriram Pemmaraju | Areas of research: Computational Epidemiology, Vaccine Allocation, Submodular Optimization
Yumna Anwar
Title: Audio-Based Cough Detection in Clinic Waiting Rooms
Abstract:
Automated cough detection has significant applications for the surveillance of diseases and supports medical decisions, as cough sounds can be a useful biomarker. However, the implementation and evaluation of robust cough detection models can be challenging due to the lack of real-world data. We make available a collection of 2,883 coughs and 3,074 non-cough sounds recorded in clinic waiting rooms that we hope will become a baseline for this task. Using this dataset, we evaluate different convolutional network architectures for classifying short audio segments as cough or non-cough. An ensemble model of convolutional neuronal networks provides the most robust performance and has a ROC AUC of 98.1%. Equally important, we construct a cough counter that incorporates the ensemble model to compute the number of coughs per day. Then, a simple linear model estimates the number of visits in which the patients report cough symptoms from the cough counts. This simple regression model can predict the number of cough visits in the clinic with an absolute mean error of 4.26 cough visits per day. Using additional information about when patients are in the clinic helps a similar regression model reach a mean absolute error of 3.65 cough visits per day. These results demonstrate the feasibility of using cough detection as a biomarker for the spread of respiratory viruses within the community.
5th year PhD student | Advisor: Octav Chipara | Areas of research: Machine learning and M-health
Ingroj Shrestha
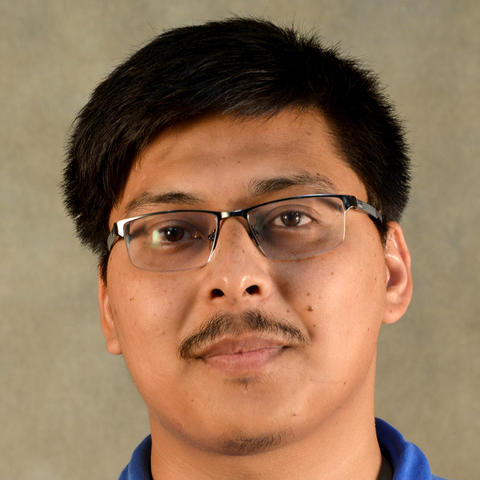
Title: Studying Bias in Large Language Models
Abstract:
Given the current generation of highly effective deep learning models trained on large data that perform well in various tasks, different companies adopt them to classify text (classification tasks). However, these systems raise social concerns by exhibiting bias against demographic group(s) (e.g., gender, race, etc.) that can disproportionately negatively impact the unfavored groups (minority). The source of bias could be categorized into three stages: (a) at the time of data sampling, which does not reflect the realistic distribution (b) during human annotation due to conscious or sub-conscious bias leading to mislabeled data (c) during model training where model might even worsen and propagate bias. We present an overview of prior research on detecting bias exhibited by a model at training time, discussing bias quantification metrics. We then highlight the gap in the research where bias is studied considering a single minority and a single majority. For example, considering race demographics, African American is the only community considered as a minority. We then present our work, which addresses this gap by studying bias from a multi-community perspective, suggesting the model-data combination community subjected to bias. [Note: abstract most closely associated with former talk title: "Understanding bias in text classification systems"]
6th Year PhD student | Advisor: Padmini Srinivasan | Areas of research: Text Mining, Natural Language Processing, and the Understanding and Mitigation of Bias
Maaz Bin Musa
Title: Unveiling the Digital Veil: Empowering Transparency and Accountability in Online Privacy
Abstract:
The internet today is powered by large amounts of user data which organizations gather either directly or indirectly. Once an organization acquires this data, the users lose all control over how this data is used and maintained. This data exchanges hands several times on the backend, without any knowledge to the user, occasionally to malicious entities. Additionally, even when the user knows who has their data, the data-rights given to the users by the government are limited and extremely complicated to understand and execute. My work focuses on: (1) increasing transparency by uncovering backend data flows and (2) increasing accountability by programmatically analyzing compliance of organizations that consume user data, under the lens of data rights regulation. To achieve transparency, I utilize personalized ads and honeytraps to uncover backend dataflows. Whereas, to increase accountability, I use NLP tools to develop text classification pipelines for compliance auditing. Overall, my goal is to re-equip users with the power to control their online data.
5th year PhD student | Advisors: Rishab Nithyanand | Areas of research: Privacy Measurement and Natural Language Processing
Keynote Speaker: Garrett Morris
Title: On Extensibility
Abstract:
This talk will introduce my research on programming languages, exemplified by some recent results on modularity and extensible data types. My research into programming languages and their type systems is guided by two overriding goals. On the one hand, types should precisely specify program behavior, allowing programmers to rule out classes of erroneous behavior. On the other, types should enable expressive tools, allowing programmers to build modular, re-usable software and software components. My work inhabits the intersection of these ideas, refining generic programming mechanisms both to better enforce intended program behavior and to support more expressive abstractions.
Extensibility is an evergreen problem in programming, and so in programming language design. The goal is simple: specifications of data should support the addition of both new kinds of data and new operations on data. Despite this problem having been identified as early as 1975, modern languages lack effective solutions. Object-oriented languages require programmers to adopt unintuitive patterns like visitors, while functional languages rely on encodings of data types. Lower-level languages, where similar problems arise, rely on textual substitution.
In this talk, I will present recent work with James McKinna and Alex Hubers which proposes a unified approach to extensible data specifications. This work generalizes existing systems for extensible data types in two ways: It captures examples that are inexpressible in all other systems and generalizes over a variety of approaches to extensibility previously thought incompatible. More recently, we have extended this approach to generic programming over extensible data types; that is to say, capturing properties and invariants of extensible data types without putting any concrete restrictions on the structure of the data.
Bio: J. Garrett Morris is an Assistant Professor and Emeritus Faculty Scholar in the Department of Computer Science at the University of Iowa. He received his PhD from Portland State University in Oregon, and post-doctoral training at the University of Edinburgh, Scotland. His research focuses on the development of type systems for higher-order functional programming languages, with the twin aims of improving expressiveness and modularity in high-level programming and supporting safe concurrent, low-level, and effectful programming. His work has been published in the top venues in theoretical programming languages, and is supported by the National Science Foundation.
Individuals with disabilities are encouraged to attend all University of Iowa–sponsored events. If you are a person with a disability who requires a reasonable accommodation in order to participate in this program, please contact in advance at