- Interests
- Types
CS Colloquium - Melding NLP and Causal Inference
Sep 8, 2023
03:30 PM - 04:30 PM
2 West Washington Street, Iowa City, IA 52240
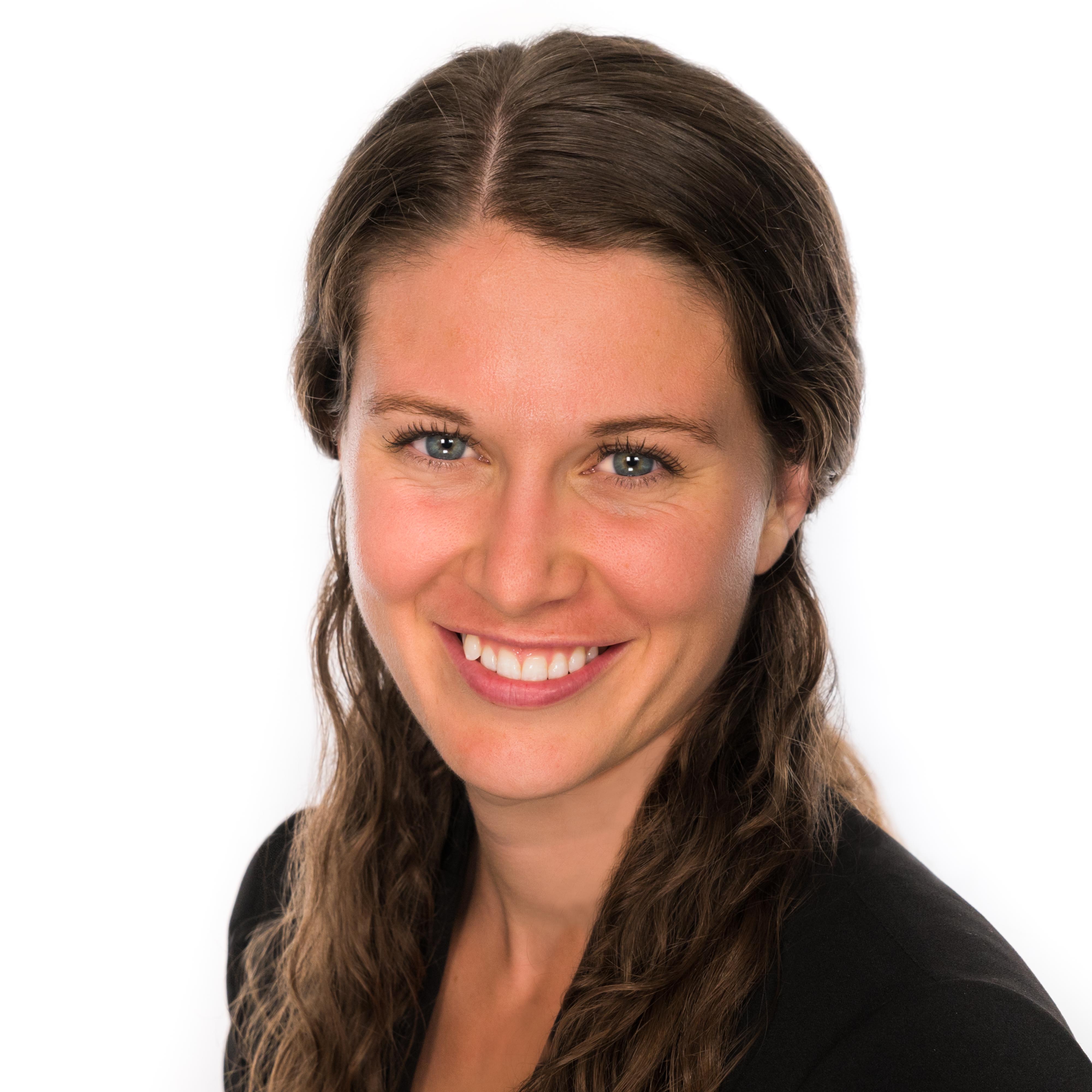
Speaker
Katie Keith
Abstract
Data-driven decision making often relies on crafting effective policies or interventions. Quantifying the effect of these interventions (real or hypothetical) is a task in the broader field of causal inference. In our modern data-rich world, these causal inference pipelines increasingly include text data, for example, from electronic health records, newspapers, search engine logs, or social media. Yet, at this intersection of natural language processing (NLP) and causal inference, there are still a plethora of open problems.
This talk will focus on several recent advances at this intersection of NLP and causal inference. First, we will explore a case study of estimating the causal effects of peer review policies from publication venues that shift policies from single-blind to double-blind from one year to the next. In this setting, the content of the manuscript is a confounding variable—each year has a different distribution of scientific content which may naturally affect the distribution of reviewer scores. To address this textual confounding, we extend variable ratio nearest neighbor matching to incorporate text embeddings. We compare this matching method to a widely-used causal method of stratified propensity score matching and a baseline of randomly selected matches. We find human judges prefer sampled matches from our method 70% of the time. Second, we will discuss an empirical evaluation approach to causal inference with text data and other high-dimensional covariates. We build on a promising empirical valuation strategy that simplifies evaluation design and uses real data: subsampling randomized controlled trials (RCTs) to create confounded observational datasets while using the average causal effects from the RCTs as ground-truth. We contribute a new sampling algorithm, which we call RCT rejection sampling, and provide theoretical guarantees that causal identification holds in the observational data to allow for valid comparisons to the ground-truth RCT. As a proof of concept, we implement an example evaluation pipeline with a novel, real-world RCT—which we release publicly—consisting of approximately 70k observations and text data as high-dimensional covariates.
Bio
Katherine (Katie) Keith is currently an Assistant Professor of Computer Science at Williams College. Her research interests are at the intersection of natural language processing, computational social science, and causal inference. Previously, she was a Postdoctoral Young Investigator with the Semantic Scholar team at the Allen Institute for Artificial Intelligence, and she graduated with a PhD from the Manning College of Information and Computer Sciences at the University of Massachusetts Amherst. She has been a co-organizer of the First Workshop on Causal Inference and NLP, the NLP+CSS Workshop, and the NLP+CSS 201 Online Tutorial Series. She also hosts the podcast Diaries of Social Data Research and was a recipient of a Bloomberg Data Science PhD fellowship.
Individuals with disabilities are encouraged to attend all University of Iowa–sponsored events. If you are a person with a disability who requires a reasonable accommodation in order to participate in this program, please contact in advance at